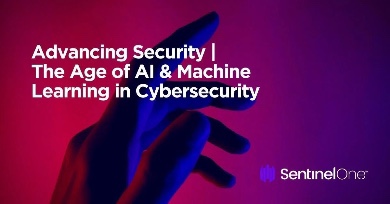
The rapid advancements in Artificial Intelligence (AI) and Machine Learning (ML) have transformed various industries, including cybersecurity. AI and ML technologies offer significant potential in automating tasks, augmenting human capabilities, and improving overall cybersecurity defenses. However, this progress comes with ethical considerations, especially concerning the impact on the cybersecurity workforce. In this blog post, we delve into the integration of AI and ML in cybersecurity workforce management, examining the ethical implications of automation and its potential effects on cybersecurity professionals.
- AI and ML in Cybersecurity
a. Augmenting Cybersecurity Capabilities:
AI and ML technologies empower cybersecurity professionals with sophisticated tools to detect and respond to threats with greater speed and accuracy. These technologies can analyze vast amounts of data, identify patterns, and predict potential attacks, enhancing overall cybersecurity posture. By leveraging AI and ML, organizations gain an advantage in their ability to defend against sophisticated cyber threats.
b. Automating Repetitive Tasks:
Routine and repetitive tasks, such as log analysis, threat hunting, and incident response, can be automated using AI and ML algorithms. Automation allows cybersecurity teams to focus on strategic and high-impact activities, improving efficiency and resource allocation. By offloading mundane tasks to AI-driven solutions, cybersecurity professionals can concentrate on more complex and creative problem-solving.
- The Ethical Implications of AI and ML in Cybersecurity Workforce Management
a. Job Displacement Concerns:
The integration of AI and ML in cybersecurity may lead to concerns about job displacement for certain roles. As tasks become automated, cybersecurity professionals may need to adapt their skill sets and transition to more complex and strategic responsibilities. Organizations should proactively address these concerns and invest in upskilling programs to prepare the cybersecurity workforce for the changing landscape.
b. Skills Gap and Training:
The implementation of AI and ML requires cybersecurity professionals to acquire new skills to operate and manage these technologies effectively. Addressing the skills gap through comprehensive training and upskilling programs becomes essential to ensure a capable and future-ready cybersecurity workforce. Organizations should invest in continuous education and professional development to equip their workforce with the necessary expertise.
c. Data Bias and Fairness:
AI and ML algorithms learn from historical data, which may contain biases and imbalances. In cybersecurity, this could lead to biased decision-making and potential disparities in threat analysis and response. Ensuring fairness and mitigating bias should be a priority in deploying AI and ML solutions. Organizations should establish guidelines for data collection and model training to minimize bias and ensure equitable outcomes.
- Balancing Automation and Human Expertise
a. Human-in-the-Loop Approach:
A human-in-the-loop approach, where AI and ML technologies support human decision-making, can strike a balance between automation and human expertise. Cybersecurity professionals remain actively involved in critical decision-making processes, ensuring ethical considerations and human oversight. By combining AI insights with human judgment, organizations can make well-informed and responsible decisions.
b. Emphasizing Human Creativity:
AI and ML excel at processing large volumes of data, but human creativity and intuition are invaluable when tackling novel and complex threats. Cybersecurity professionals can leverage AI insights to develop innovative strategies and responses to emerging cyber risks. By fostering a collaborative environment that encourages creativity, organizations can harness the power of human intellect alongside AI capabilities.
- Building Trust and Transparency
a. Explainable AI (XAI):
Explainable AI (XAI) is crucial in the cybersecurity context, where the “black box” nature of AI algorithms can raise concerns about transparency. XAI techniques provide interpretable explanations for AI-driven decisions, fostering trust between cybersecurity professionals and AI technologies. By promoting transparency, organizations can ensure that AI-driven decisions are understandable and accountable.
b. Clear Communication:
Organizations must communicate transparently with their cybersecurity workforce about the implementation of AI and ML technologies. Open discussions about automation’s goals, limitations, and potential workforce impact can alleviate concerns and foster collaboration. By engaging cybersecurity professionals in the decision-making process, organizations can ensure that ethical considerations are prioritized.
Conclusion
The integration of AI and ML in cybersecurity workforce management offers great promise in bolstering cybersecurity defenses and improving overall efficiency. However, ethical considerations are paramount when deploying these technologies to ensure a harmonious coexistence between automation and human expertise. Striking a balance between AI-driven automation and human creativity, addressing job displacement concerns through upskilling, and mitigating biases are essential steps in navigating the ethical implications of AI and ML in cybersecurity. By fostering transparency, building trust, and investing in workforce development, organizations can build a strong and resilient cybersecurity workforce that embraces AI and ML advancements responsibly, ensuring a secure digital future. Through ethical AI and human collaboration, cybersecurity professionals can continue to protect organizations from cyber threats effectively, creating a safer digital landscape for all.