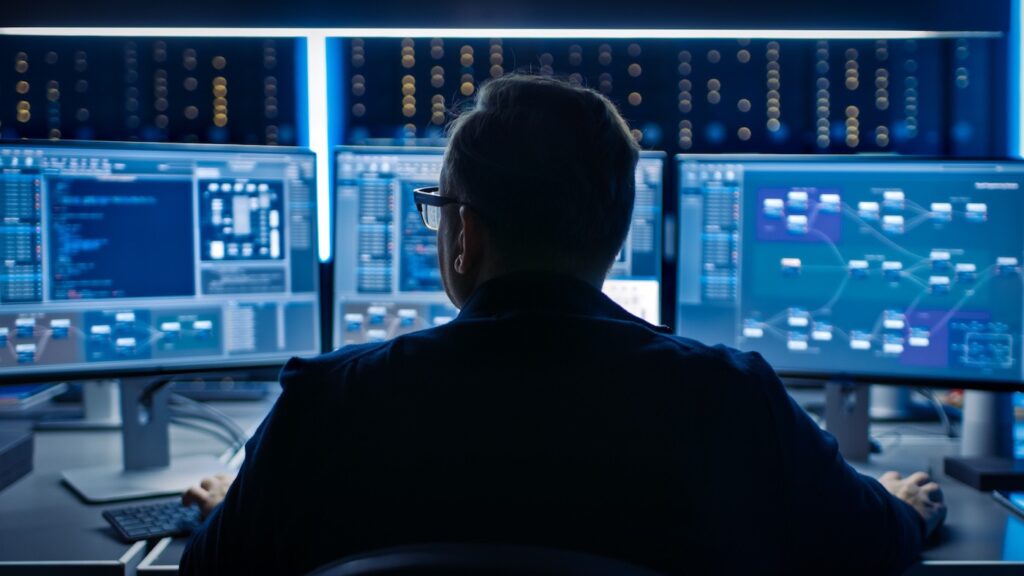
Organisations are increasingly turning to ML-based cybersecurity systems to detect and respond to changing threats as machine learning (ML) continues to revolutionise the cybersecurity landscape. While these technologies have sophisticated capabilities, they also create serious privacy problems, especially when dealing with sensitive data. We dig into the various privacy issues that arise when employing machine learning models in cybersecurity operations in this blog post. By understanding these risks, organizations can strike a balance between robust security measures and protecting individuals’ privacy.
- The Role of Machine Learning in Cybersecurity
Machine learning has become a game-changer in cybersecurity, empowering systems to detect anomalies, classify threats, and adapt to new attack vectors with unmatched speed and accuracy. ML models leverage vast amounts of data, including sensitive information, to improve threat detection and enhance overall cybersecurity resilience. However, the use of such data introduces various privacy implications that demand careful attention.
- The Intersection of ML and Data Privacy
a. Data Collection and Storage:
ML-based cybersecurity systems require substantial datasets for training and continuous learning. However, the collection and storage of sensitive data raise concerns about data breaches and unauthorized access. Organizations must implement robust security measures and data encryption techniques to safeguard the confidentiality and integrity of this information.
b. Anonymization and De-identification:
Organizations must carefully anonymize or de-identify data before processing it through ML models. Failure to do so could lead to unintended disclosure of sensitive information. Techniques like differential privacy can help preserve privacy while still allowing the data to be useful for training the models.
c. Informed Consent:
User consent becomes essential when handling personal or sensitive data. Organizations must inform individuals about data collection, usage, and the implications of sharing their information. Obtaining explicit consent from users ensures transparency and trust in data processing activities.
- Potential Privacy Risks in ML-Based Cybersecurity
a. Data Exposure:
If appropriate privacy measures are not in place, the usage of sensitive data in ML-based cybersecurity systems can result in data exposure. A data breach can have serious implications, including financial loss and reputational harm. Organisations must audit and monitor their systems on a regular basis in order to detect and remedy any risks.
b. Model Inversion Attacks:
Cyber attackers may attempt model inversion attacks to infer sensitive data from the output of ML models. For instance, an adversary could try to deduce private information from the model’s predictions. Employing adversarial training techniques can help mitigate such attacks and enhance the privacy of the ML model.
c. Membership Inference Attacks:
Attackers could exploit ML models to determine whether a particular data point was used during training. This poses a risk to data privacy, especially when dealing with individual-level data. Employing privacy-preserving techniques like federated learning can reduce the risk of membership inference attacks.
d. Bias and Discrimination:
ML models can inherit biases from the training data, leading to discriminatory outcomes in cybersecurity operations. This raises ethical concerns and affects privacy when certain groups are disproportionately targeted or ignored. Regularly auditing the training data for bias and using fairness-aware algorithms can help address this issue.
- Mitigating Privacy Risks in ML-Based Cybersecurity
a. Data Minimization:
Adopting a data minimization approach involves collecting and retaining only the necessary data for model training, reducing the risk of exposing sensitive information. This approach ensures that data used in ML models is limited to what is required for effective cybersecurity operations.
b. Secure Data Sharing Protocols:
Implementing secure data sharing protocols, such as differential privacy, can enhance data privacy by adding noise to the data before training the model. This ensures that individual data points are not distinguishable in the model’s training data.
c. Federated Learning:
Federated learning allows ML models to be trained across multiple devices without centralizing sensitive data, reducing the risk of data exposure. This approach is particularly beneficial when dealing with data from different sources, such as various cybersecurity organizations.
d. Explainable AI (XAI):
Organisations can utilise XAI to provide explanations for model outputs, ensuring a transparent and responsible decision-making process.
- Legal and Ethical Considerations
a. Regulatory Compliance:
Organizations must adhere to relevant data protection regulations, such as GDPR or CCPA, to ensure the lawful processing of personal data. Staying compliant with these regulations not only protects individuals’ privacy but also helps avoid legal penalties.
b. Ethical AI Guidelines:
Developing and following ethical AI guidelines ensure responsible and privacy-conscious use of ML-based cybersecurity systems. Adopting ethical guidelines encourages organizations to prioritize privacy and fairness in their AI practices.
Conclusion
The adoption of machine learning in cybersecurity has transformed how organizations defend against cyber threats. However, this transformation comes with significant privacy concerns when handling sensitive data. By carefully assessing potential risks and implementing privacy-preserving measures, organizations can strike a balance between advanced security capabilities and protecting individuals’ privacy. Emphasizing data minimization, secure data sharing protocols, and explainable AI can foster transparency and trust while navigating the complexities of ML-based cybersecurity. Ultimately, a privacy-first approach will help build robust and ethical cybersecurity practices that safeguard both data and individuals’ rights in the digital age.